
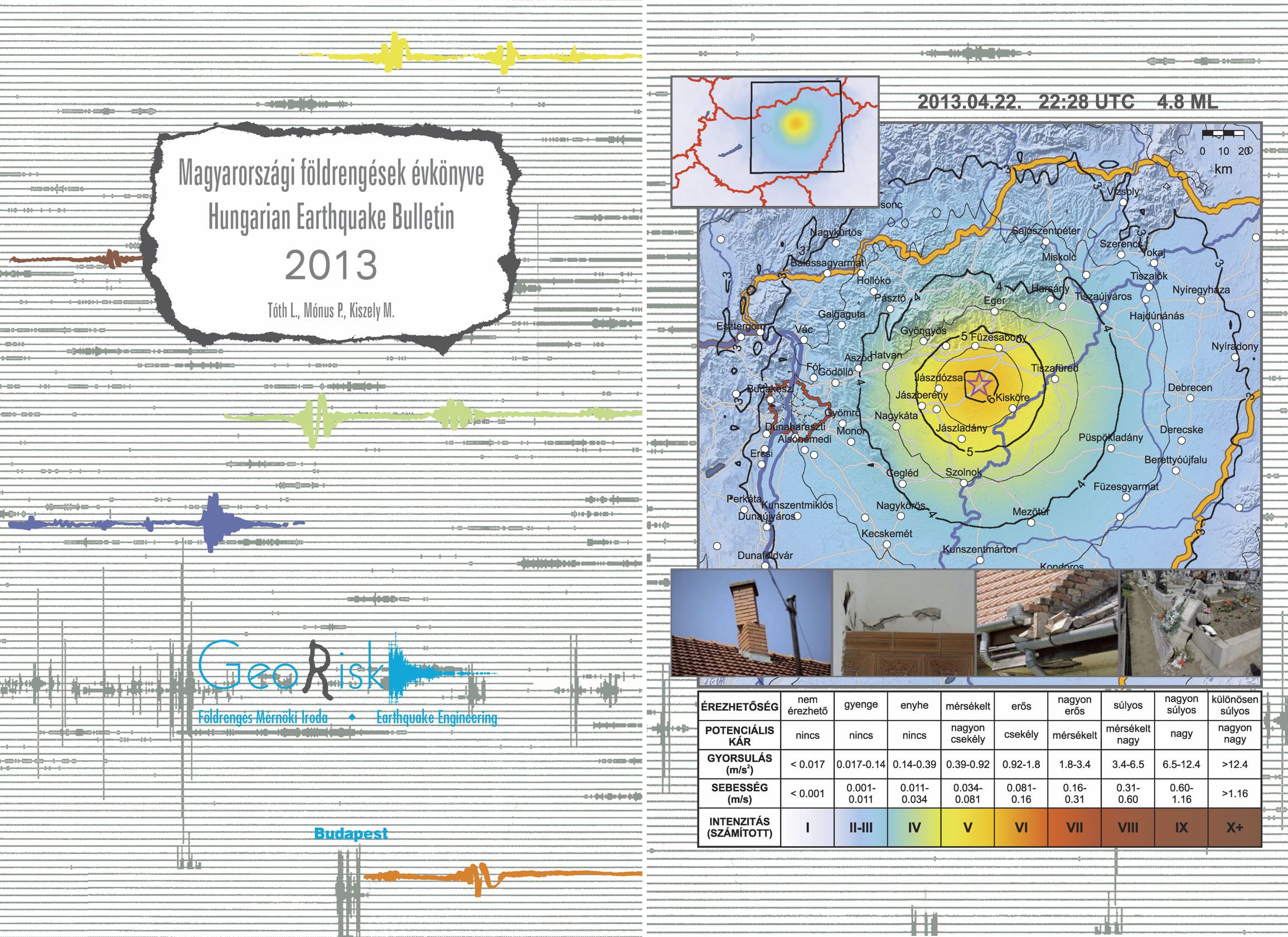
The horizontal axis is the difference between manually labeled sampling points and the sampling points picked up by the deep learning model.įigure 6. Examples of randomly inspected samples with large differences between the manual labeling of the DiTing dataset and the automatic detection results of PhaseNet.įigure 7. Histograms of the theoretical traveltime residuals for manually picked P- and S-wave arrival times.Bulletin of Earthquake Engineering presents original, peer-reviewed papers on research related to the broad spectrum of earthquake engineering.
#Lithuania earthquake bulletin serial number#
The blue lines are labels of P-wave arrival times the red lines are labels of S-wave arrival times the upward and downward arrows denote the “positive” and “negative” P-wave first-motion polarities, respectively the waveforms are arranged by epicentral distance the serial number is in the “seismic event number.station number” format, which is the unique number of the waveform in the DiTing dataset.įigure 5. Results of benchmark tests for the DiTing dataset using the EQTransformer and PhaseNet algorithms. The orange line is the tectonic line.įigure 2. Histograms of the epicentral distance (a), magnitude (b), signal-to-noise ratio of P-waves (c), and signal-to-noise ratio of S-waves (d) in the DiTing dataset.įigure 3. Distributions of back azimuth (a), P-wave first-motion polarity (b) (where “U” represents upward, “D” represents downward, and “I,” “E,” and “-” represent impluse, emergent, and uncertain first-motion labeling features, respectively), and magnitude type (c) for the DiTing dataset.įigure 4. Examples of labeled waveforms at epicentral distances of (a) 0–160 km and (b) 160–330 km. The dots denote earthquakes, with the color indicating the earthquake depth. Such research will further promote the development and application of artificial intelligence in seismology.įigure 1. Distribution of the earthquakes (a) and seismic stations (b) included in the DiTing dataset. The dataset compiled in this study can serve as a high-quality benchmark for machine learning model development and data-driven seismological research on earthquake detection, seismic phase picking, first-motion polarity determination, earthquake magnitude prediction, early warning systems, and strong ground-motion prediction. The magnitudes of seismic events, epicentral distance, signal-to-noise ratio of P-wave data, and signal-to-noise ratio of S-wave data ranged from 0 to 7.7, 0 to 330 km, –0.05 to 5.31 dB, and –0.05 to 4.73 dB, respectively. Each three-component waveform contained a considerable amount of descriptive information, such as the epicentral distance, back azimuth, and signal-to-noise ratios. All waveforms were sampled at 50 Hz and cut to a time length of 180 s starting from a random number of seconds before the occurrence of an earthquake.

The obtained dataset included 2,734,748 three-component waveform traces from 787,010 regional seismic events, the corresponding P- and S-phase arrival time labels, and 641,025 P-wave first-motion polarity labels. Data were recorded using broadband and short-period seismometers. In this study, based on the 2013–2020 seismic cataloging reports of the China Earthquake Networks Center, we constructed an artificial intelligence seismological training dataset (“DiTing”) with the largest known total time length. Vast amounts of high-quality labeled data are required to develop and apply artificial intelligence in seismology research. In recent years, artificial intelligence technology has exhibited great potential in seismic signal recognition, setting off a new wave of research.
